Blog
Dec 6, 2024
Automation is great—until it isn’t. Google’s Performance Max and Meta’s Advantage+ campaigns are powerful tools, but they often leave performance marketers wondering: What’s really driving results? If you’ve been struggling to understand, optimize, or trust the data, this guide will help you master your audit process.
Campaign automation tools like Google's PMax and Meta's Advantage+ allow campaigns to be optimized by the platforms themselves, benefiting from their superior infrastructure and access to data, without the need for human oversight. These tools can experiment more exhaustively with audience settings and placements, faster, than a media buying team.
However, there is a conflict of interest between the ad platforms and brands.
The conflict of interest
This AI automation comes at the cost of transparency. These tools have limited targeting options and provide only broad reporting, creating a dilemma for brands. Whereas an advertiser's objective is to maximize ROAS for every dollar deployed, an automated tool's goal is to maximize total budget deployed so long as the advertiser's ROAS requirements are met.
If an automated tool deploys some portion of a brand’s budget wastefully but ultimately meets the advertiser's performance goals, the advertiser is objectively better off as a result of the campaign. Should an advertiser care that money was spent wastefully - on inappropriate audiences or formats - if its overall ROAS performance goals are met?
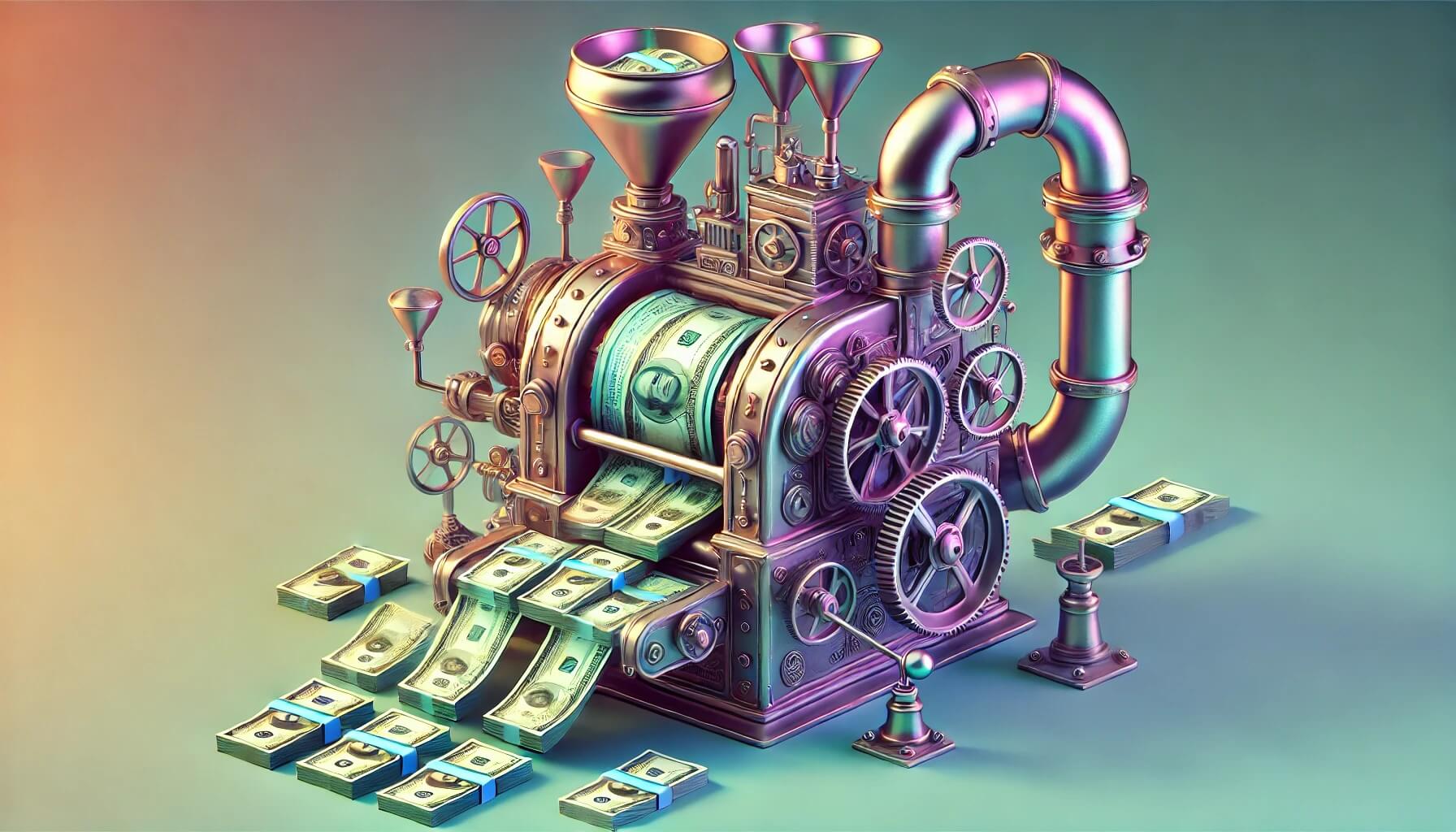
The risks of AI campaigns
This conflict of interest leads to risks and drawbacks:
1/ Little transparency - there is limited control over targeting and reports lack granular details. These campaigns feel like a black box to a large extent.
Google seems to be addressing this point given the recent updates on more insights and controls for AI-powered campaigns
2/ Incremental, profitable growth OR cannibalization? - to what extent these campaigns drive incremental, profitable growth is often a question mark. Campaigns like PMax are built to maximize your conversions value. However, marketers have complained that they also target "warm" traffic that hasn't converted yet - e.g., "past buyers" who are in another buying cycle.
3/ Impact on unit economics - automated changes in targeting are likely to affect CLV and unit economics. Little control over the mix of new and existing users also leads to potential cannibalization with CRM efforts.
The most common pitfalls
While automation promises to streamline marketing workflows and increase efficiency, many performance marketers fall into a dangerous trap: overlooking the fundamentals and how these campaigns actually work.
These are the most common pitfalls:
1/ Sub-optimal budget allocation based on platform ROAS - platform ROAS will always look great for PMax and Advantage+ campaigns. Many marketers rely blindly on these metrics, instead of analyzing backend data - incrementality and attribution;
2/ Worse unit economics - ignoring profitability metrics like POAS and CLV might lead to worse unit economics. For example, teams have seen ASC+ skewing traffic to older audiences in a way that breaks unit economics (e.g., story shared by Eric Seufert);
3/ Wasted budget with existing customers - these campaigns will likely capture more of the existing users, leading to wasted spend (CRM campaigns would already capture a big share);
4/ Blindly trust automation - overlooking rigorous auditing for audience selection and other key dimensions, which may break unit economics.
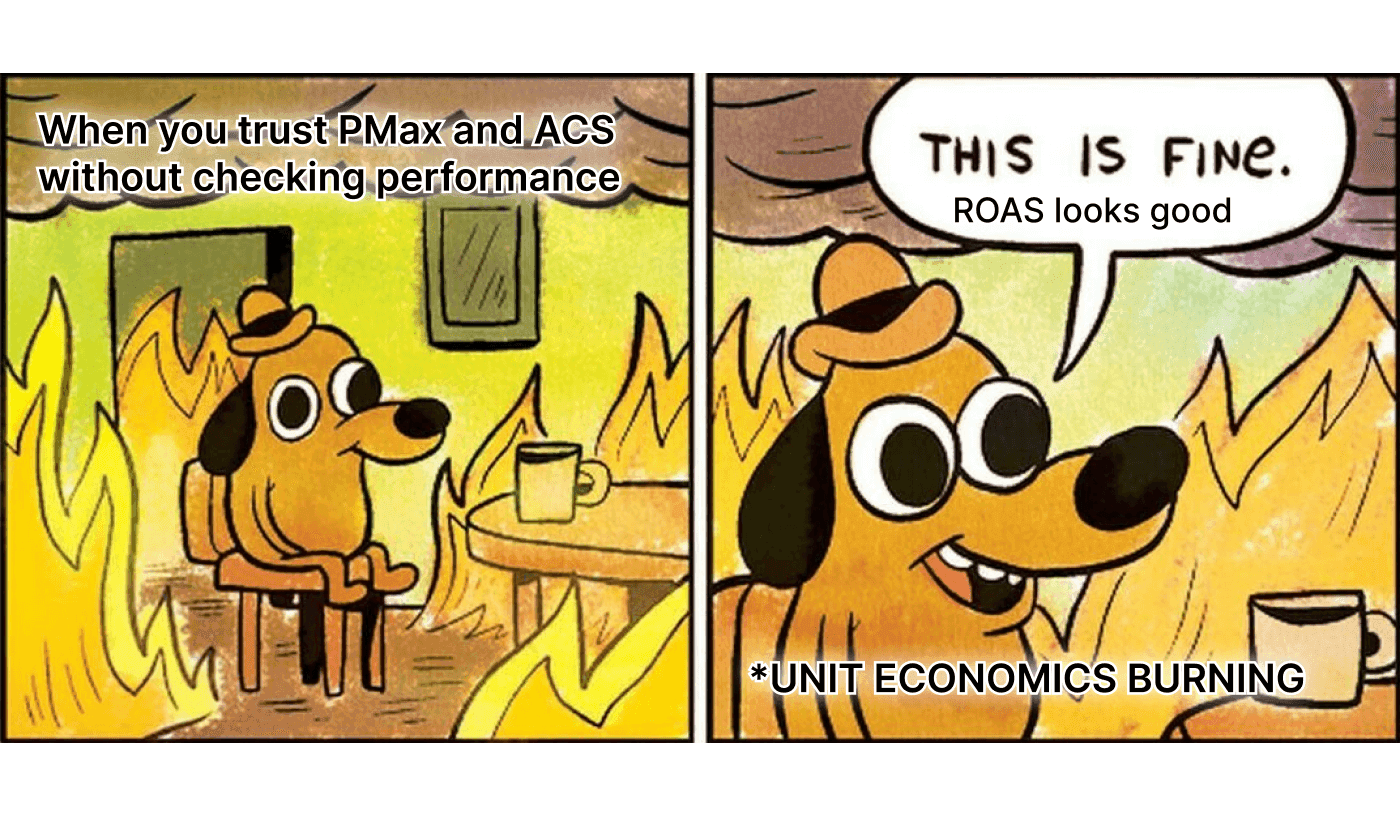
How to audit AI campaigns
Assessing the true impact of AI campaigns requires proper measurement and auditing. These are the 4 key principles:
1/ Account level testing based on internal attribution and incrementality - measure the incremental effects of AI vs traditional campaigns based on your internal measurement methods (i.e., ignore platform metrics). Rely on multiple "sources of truth";
2/ Analyze full funnel performance and mix between new and existing users - look into CLV and POAS to assess the profitability impact of such AI campaigns and track the mix between new users vs existing users. You should review this on your weekly business reviews;
3/ Audit and monitor key metrics and dimensions - keep track of targeting, audience, demographics, device, platform and other key metrics and dimensions to avoid surprises. Compare these dimensions across AI and traditional campaigns;
4/ Measure cannibalization effects - monitor very closely AI vs traditional campaigns on a very granular way to identify early on cannibalization effects.
Key takeaways
AI campaigns promise automation and efficiency but create a conflict between ad platform's efficiency and brand-specific goals.
Limited transparency means marketers have minimal insight into how their budgets are being deployed.
These campaigns risk cannibalizing existing marketing efforts rather than driving truly incremental, profitable growth.
Platform metrics can be misleading - always compare them against internal attribution and take into account profitability metrics and the mix between new and existing users.
Continuous testing, rigorous auditing, and strategic human oversight are crucial for success.
Read more on similar topics
See Clarisights in action
Curious to see how leading enterprise marketing teams go from questions to insights in minutes?
Book some time with us.