Blog
Dec 3, 2024
Marketing or Mix Modeling (MMM) is on everyone’s agenda. Its popularity has increased rapidly over the last few years. The signal loss caused by regulations like GDPR and Apple’s App Tracking Transparency (AAP) policy has been a major driver.
However, most MMM projects fail. Despite its popularity, many organizations struggle to build trust in their MMM approaches and drive tangible results. As a result, confidence in CMOs is lost and marketing strategies completely pivoted due to faulty MMM projects.
Working closely with leading marketing and data teams at organizations like Uber, Delivery Hero, and HelloFresh, we’re sharing learnings and best practices from their measurement journeys.
What is MMM
At its core, Marketing Mix Modeling is a statistical analysis technique designed to quantify the impact of various marketing activities on business outcomes. By analyzing historical data, MMM attempts to unpack the complex relationships between marketing investments and sales performance, providing insights into channel effectiveness and budget allocation.
The math and statistics behind MMM models are complex but are out of scope of this article.
While building a model, either in-house or via MMM vendors, is relatively straightforward, turning it into effective decisions is quite hard. Let’s unpack the common pitfalls.
Critical pitfalls in MMM
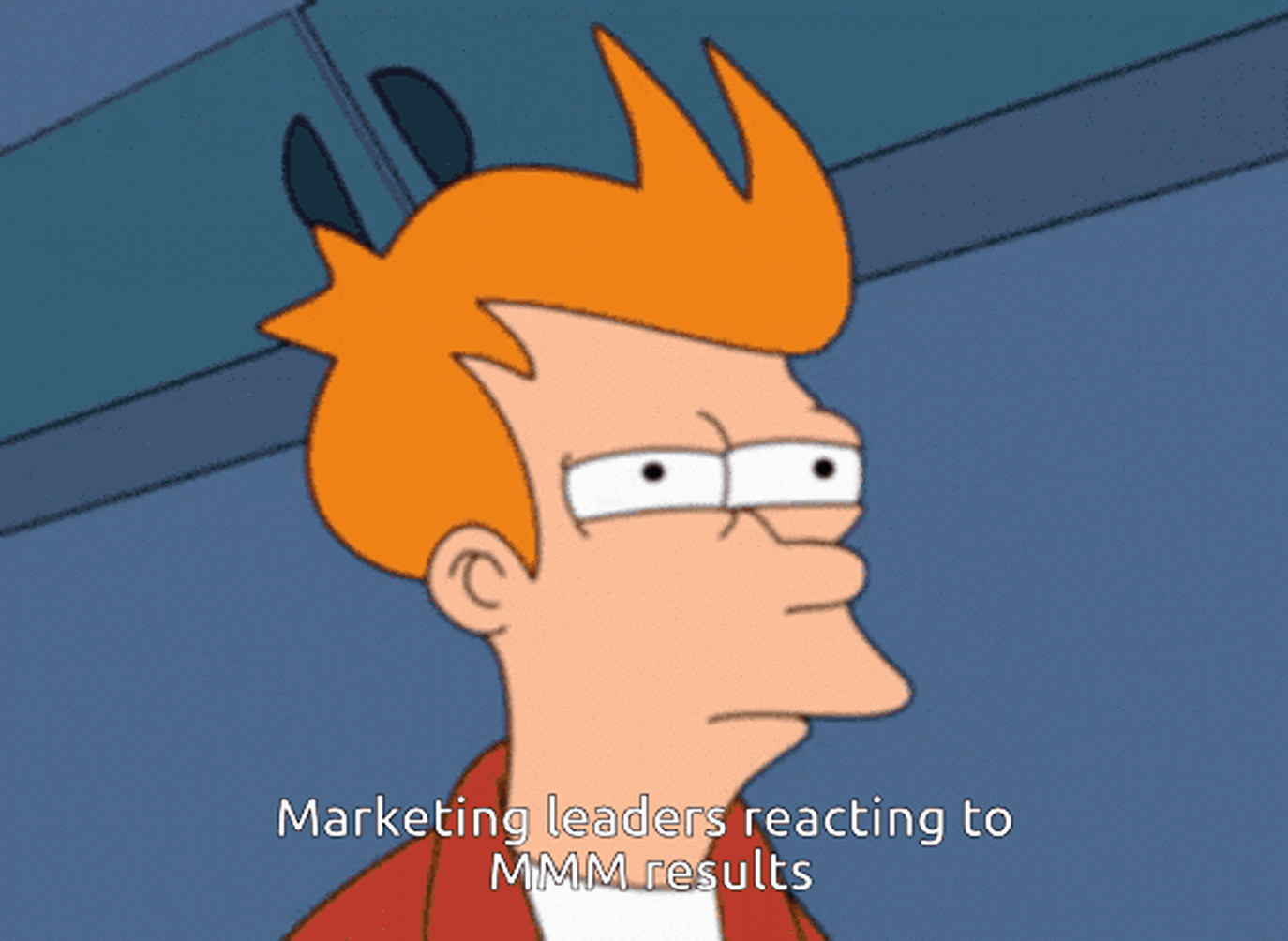
The road to effective MMM is lined with significant challenges that can compromise its value:
1/ Trust and Validation Challenges - the most significant hurdle is the lack of transparent validation mechanisms. Management teams often struggle to trust MMM outputs due to the opaque nature of the modeling process. Without a clear method to verify the model's accuracy, skepticism can quickly undermine the entire analytical effort.
2/ Limited Predictive Accuracy - MMM models typically rely on historical data, which may not accurately reflect rapidly changing market dynamics. The digital marketing landscape evolves at an unprecedented pace, making historical patterns less reliable for future predictions.
3/ Oversimplification of Marketing Interactions - traditional MMM approaches often fail to capture the nuanced interactions between different marketing channels. The complex, non-linear nature of modern marketing ecosystems cannot be fully represented by linear modeling techniques.
4/ Data Integration and Quality Challenges - collecting and harmonizing data across multiple platforms presents significant technical and methodological challenges. Inconsistent data sources, varying attribution models, and incomplete data can introduce substantial biases into the analysis. Read more here about how to overcome such challenges.
Best practices: a holistic measurement approach
To overcome these limitations, marketing and data teams are adopting a more comprehensive measurement strategy:
1/ Triangulated Measurement - instead of relying solely on MMM, successful organizations are combining multiple analytical approaches:
Marketing Mix Modeling
Multi-Touch Attribution (MTA)
Geo-Lift Testing
Incrementality Experiments
2/ Validation and transparency - implement a rigorous validation process that includes:
Preliminary model validation
Continuous performance monitoring
Cross-referencing with alternative measurement methods
Transparent reporting of model limitations and assumptions
3/ Adaptive and iterative approach - treat MMM as a dynamic, evolving process rather than a one-time solution. Continuously refine models, incorporate new data sources, and remain open to adjusting methodologies.
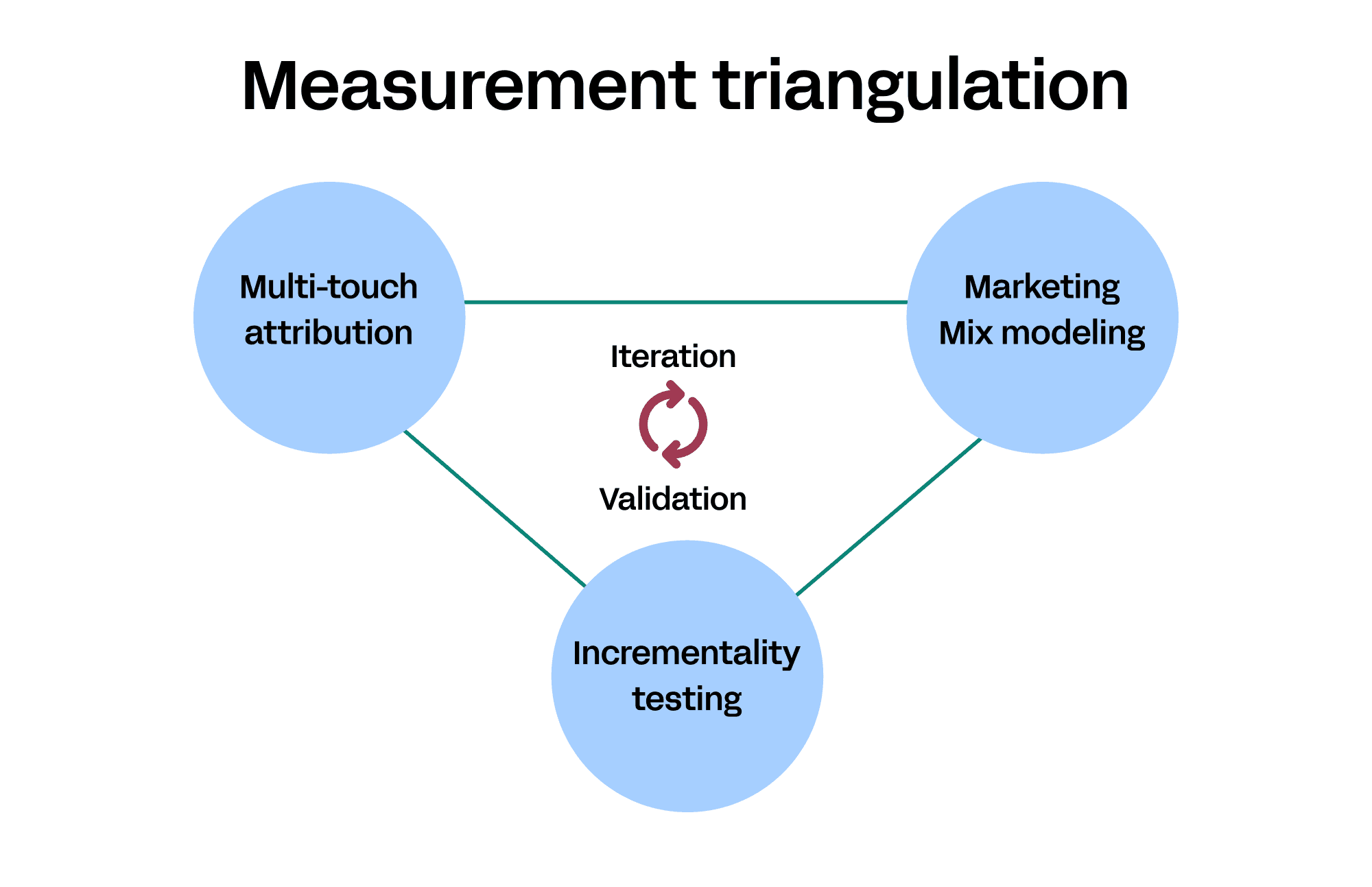
Practical implementation strategies
1/ Set clear expectations - communicate the limitations and potential of MMM to stakeholders. Position it as a directional tool rather than an absolute source of truth.
2/ Build a comprehensive measurement framework - develop a multi-layered approach that combines different analytical techniques to provide a more robust understanding of marketing performance.
3/ Automate/outsource the data collection and preparation - leverage 3rd party vendors to get harmonized data exports, ensuring reliable data and enabling the data team to focus on model building and validation vs data preparation.
4/ Benchmark internal vs external MMM models - build your internal MMM model and benchmark it against external vendors offerings. This will help you calibrate any models and build trust on any MMM results.
Key Takeaways
MMM is a not a bullet-proof solution, but rather an imperfect analytical approach
No single measurement method provides a complete picture
Combine multiple measurement techniques for more reliable insights
Prioritize transparency and continuous validation
Maintain an adaptive and experimental mindset
Avoid sharing results too early with C-level
Read more on similar topics
See Clarisights in action
Curious to see how leading enterprise marketing teams go from questions to insights in minutes?
Book some time with us.